We have reached a juncture where the retail sector must be very careful when choosing locations for its physical stores. The rules of the game have changed drastically in just one year, with a greater role for sales channels other than physical stores, albeit the former influence the latter. Against this backdrop, geographic data helps us to make this decision more manageable and with less margin for error. How is this achieved? This article answers this question.
What is retail network optimisation?
We can start with the most basic methodology, which is based on the study of critical points that historically and currently have high levels of traffic and congestion, reinforcing them.
By retail network optimisation we mean the process of optimising all aspects of retail operations, such as: location, store format and size:
- Location, format and size of stores.
- Products offered in each store.
- Cannibalisation levels.
- Effects as a result of omnichannel.
- Installation of order pick-up points.
- Etc.
With retail network optimisation, the best possible synchronisation is achieved among the various players involved within the company. We thus avoid undesirable situations, such as cannibalisation between stores. This is all done with a leading role for different data streams that provide high-value geolocalised information.
Why use quality data streams for a guaranteed retail network optimisation?
Broadly speaking, we may say that retail network optimisation provides businesses in the sector with 3 major competitive advantages:
- It reduces the risk of failure when planning the geographic distribution of stores, designing the product portfolio of each store or strategies to avoid cannibalisation, or sales drops due to having several channels.
- It offers much more accurate profit forecasts, both for existing stores and for those that are planning to open.
- It can understand the environment and detect opportunities much more quickly in the complex scenario described below.
All this in an environment in which omnichannel has become the dominant trend, especially in the wake of the change in consumer behaviour patterns caused by the COVID-19 pandemic.
The fact is that the presence or absence of physical stores in certain locations can affect online sales in one way or another, and vice versa. On the one hand, having e-commerce that meets customer requirements can reduce visits to physical stores; on the other hand, customers may prefer to “see” the product in person before purchasing it online.
We can also highlight the so-called halo effect, which shows up in markets where there is a significant difference between actual and potential sales. For example, cities in which a business has a significant and stable volume of online sales and few physical stores. In these cases, profits can grow significantly if stores are opened there.
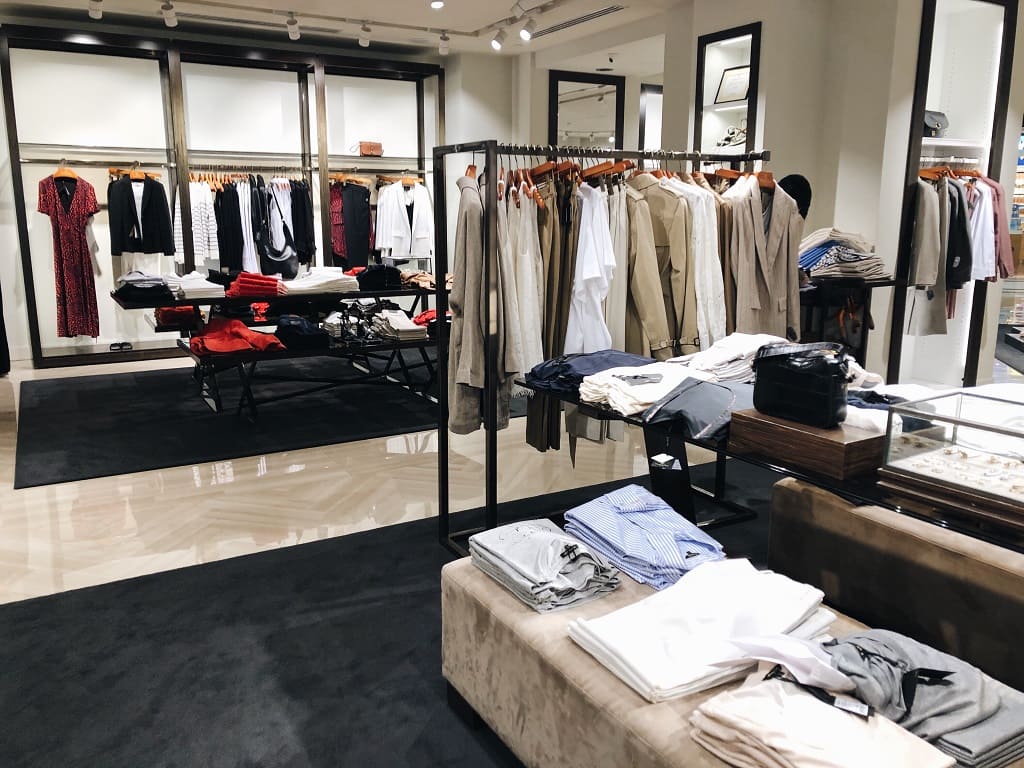
Nor should we forget about cannibalisation and its effects. Cannibalisation can occur between stores of the same type or between different channels, such as online stores or outlets versus full-price stores.
These omnichannel effects on business results remain an outstanding matter for many retail companies. In fact, there are still many that only analyse the sales figures to study these effects, extrapolating their conclusions to the rest of the markets, without considering the particularities of each and other equally- or more-influential factors.
This increases the risk of suffering consequences such as failed investments with results that can be fatal for the future of the business.
To avoid such situations, retail network optimisation works with geospatial data and artificial intelligence to provide an accurate view of the impact of the presence, opening and closing of stores on the company’s entire ecosystem, including the online factor.
Moreover, they offer knowledge on the areas for “attracting” customers, the capacity of each market or the maximum number of establishments it is capable of hosting, cannibalisation levels, etc.
Thus, managers can make better decisions regarding expanding and restructuring their networks by opening new stores or closing or relocating others. They can also decide what types of products or services to offer in each store.
Main data streams used in retail network optimisation
We have already mentioned that none of this would be possible without data, especially that with a geographic component. But what are the most common data streams used for retail network optimisation?
Firstly, we may mention the company’s internal data in terms of sales, in-person assistance to stores, e-commerce visits, greater and lesser successful products, among many others.
This data shows greater potential if we cross reference it with information of various kinds on the locations being studied, such as:
- Socio-demographic aspects: average age, income levels, types of families, education, unemployment rates, crime rates, etc.
- Consumption habits: do they prefer online or in-store shopping? What are their preferred days and times of day for shopping? What kind of products do they prefer? What is the average expenditure per purchase?
- Mobility patterns: do they usually travel by private car or by public transport? What are their most common routes? Do they prefer to shop on foot? Do they shop near their workplace or in the vicinity of their home?
- Data on the real estate market of the premises and plots to be analysed.
- Analysis and influence of the presence of points of interest, such as nearby establishments, either belonging to the same company or to the competition.
- Satellite images that facilitate the cartographic representation of the conclusions drawn.
Artificial intelligence algorithms working alongside these data streams provide the answers to everything we need to know to make our retail network optimisation a success. The performance figures of the shops will bear this out.
If we have convinced you of the usefulness of this method, at Locatium we have everything you need to plan and implement it. Shall we start this journey now? Contact us, we are keen to know more about your project.